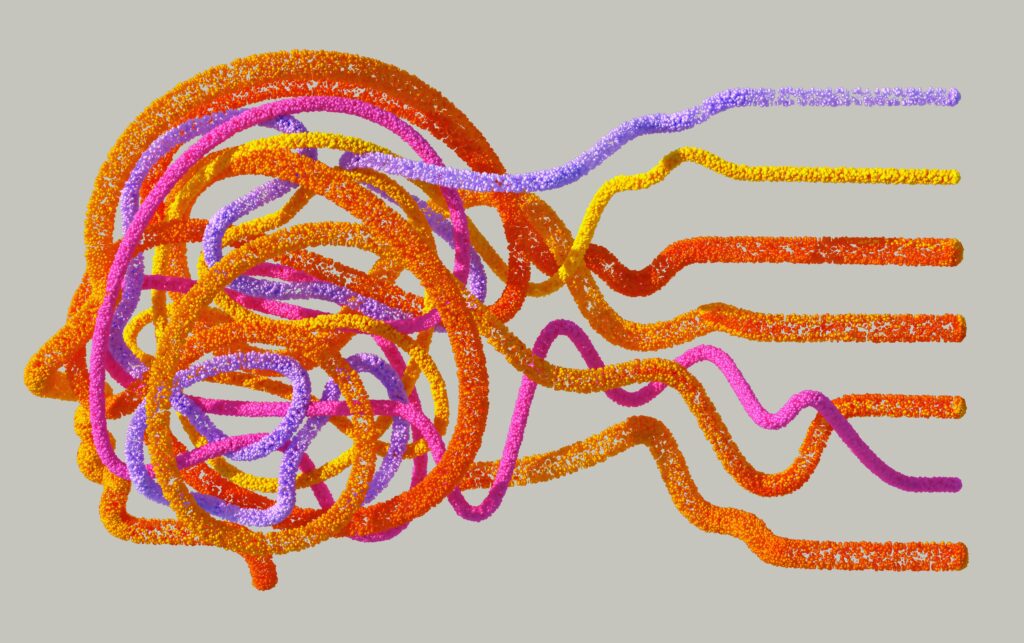
Recent advancements in AI have led to transformative applications across diverse fields. AI-driven healthcare tools are enhancing diagnostic accuracy and drug discovery. Identifying the fundamental origins of illnesses remains a significant hurdle in human genetics. With countless potential mutations and limited experimental information, it remains largely complicated which mutations may lead to diseases.
A missense mutation is a genetic disease where a single nucleotide change in the DNA sequence results in a different amino acid in the protein. Due to a different amino acid being translated, this alteration will often affect the function and structure of the protein. However, while the average human carries more than 9000 missense mutations, most have little to no effect or are “benign”, but others can severely affect protein functions or are “pathogenic”. A missense mutation that has been identified can be used in the diagnosis of genetic disorders, such as sickle cell disease, and type 2 diabetes.
Categorizing missense mutation is an important step to understanding which of these alterations in proteins may be linked to diseases. Out of over 4 million observed missense mutations in humans, only 2% have been labeled as “pathogenic or “benign” by experts. The remaining are largely considered insignificant due to a lack of experimental or subject data. The absence of accurate missense mutation functional predictions limits the diagnostic rate of rare diseases, as well as the development of clinical treatments that address underlying genetic causes.
Experiments to identify diseases causing mutations can be both costly and time-consuming. Every protein is distinct, which requires designing individual experiments that can span months. Artificial Intelligence models can close that gap by exploiting patterns in biological data to predict the pathogenicity of unannotated mutations. Leveraging AI predictions, researchers can obtain preliminary results for multiple proteins simultaneously, aiding in the allocation of resources and expediting research endeavors.
AlphaMissense is built upon Google DeepMind’s revolutionary AlphaFold2, which predicts protein structure based on amino acid sequences. AlphaMissense is able to predict mutation pathogenicity from features derived from the amino-acid sequence and outputs a score between 0 and 1 approximately rating the likelihood of a mutation being pathogenic. AlphaMissense combines three layers of reasoning to train the model.
First, AlphaMissense uses weakly supervised learning, where pathogenicity is weakly labeled either “benign” or “pathogenic” mutations depending on how frequently observed or hypothetical unobserved the mutation is in the human population, respectively. Second, AlphaMissense is trained unsupervised, which means there was no prelabelled mutation information. Alpha Missense was tasked to model the distribution of amino acids at a given sequence position to find the co-variance between mutation and amino acid sequence. Third, AlphaMissense leverages protein structure context from a missense mutation to determine its pathogenicity. AlphaMissense does not predict differences in protein structure of missense substitutions, but rather, unaltered structural predictions are used to guide the model. If the structure is vastly different between missense mutation and unaltered, then there is likely a “pathogenic” mutation, and vice versa a “benign” mutation.
AlphaMissense was incredibly successful yielding a 90% precision across disease mutation databases and experimental benchmarks. AlphaMissense outperformed other computational programs in the field of categorizing missense mutations, such as ClinVar. Due to the high successful yield, it is reasonable to presume high accuracy in its categorization of 89% of all 71 million possible missense mutations as either likely pathogenic or likely benign. By contrast, only 0.1% of all 71 million possible missense mutations have been confirmed by human experts.
While the predictions of AlphaMissense are not designed for clinical diagnosis, it serves as a good reference point along with other patient symptoms to advance rare genetic disease diagnosis. It is like the genetic test boxes from 23andMe which serve as an indicator prompting individuals to consult with healthcare professionals for a comprehensive assessment. AlphaMissense advanced the field by analyzing mutation pathogenicity that would have been otherwise impossible to do experimentally, due to time and cost. This innovation has the potential to significantly impact our understanding of genetic diseases and the development of new health product solutions.